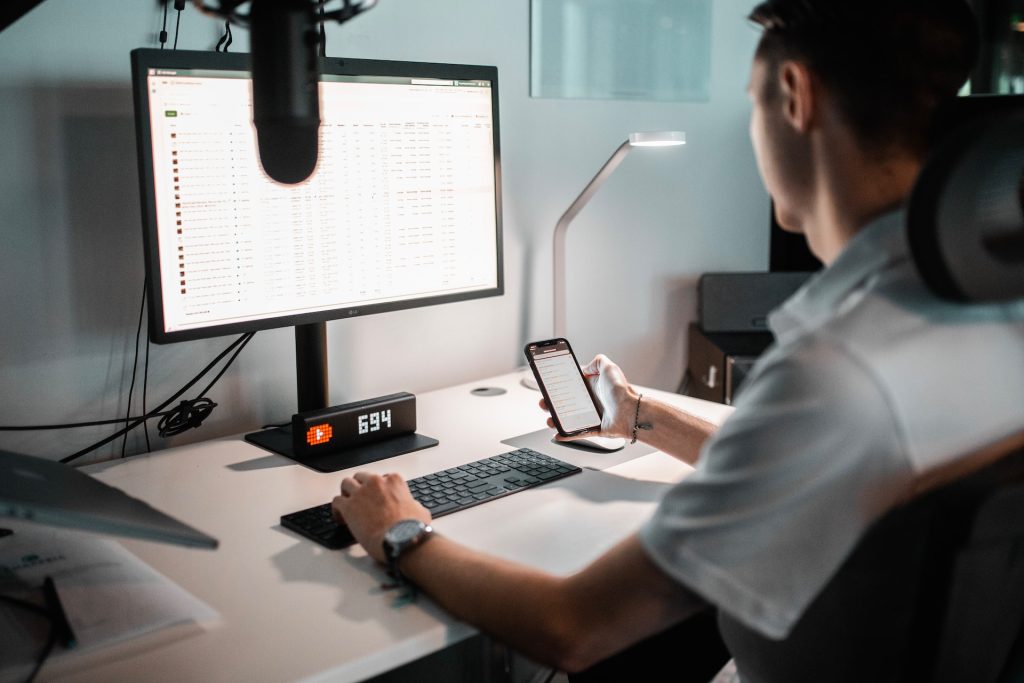
Pic Courtesy - UnSplash
Artificial intelligence (AI) has transformed many aspects of modern business operations, particularly predictive management. Its integration into business processes has fundamentally altered how industries approach maintenance, downtime reduction, and fault detection. AI-powered predictive analytics has helped businesses streamline operations, optimize maintenance schedules, and improve overall efficiency.
Reduction of Downtime
One of the most important benefits of AI in predictive management is the significant reduction in downtime. AI can forecast potential issues and address them proactively before they become major problems by combining historical data, real-time information, and predictive algorithms. This proactive approach reduces unexpected breakdowns and unplanned downtime, ensuring uninterrupted operations and maximum productivity.
Case Study : Rolls-Royce | Industry: Aerospace
- Challenge: Unscheduled downtime of aircraft engines was a major cost driver for Rolls-Royce, resulting in lost revenue and customer dissatisfaction.
- Solution: Rolls-Royce implemented an AI-powered predictive maintenance system that analyzed engine data in real time to detect potential issues and predict failures before they occurred.
- Benefits:
- Reduced unplanned downtime by 30%
- Saved $250 million annually
- Improved customer satisfaction
Optimization of Maintenance Schedule:
AI analyzes data patterns to predict when equipment will require maintenance. This foresight enables businesses to optimize maintenance schedules, avoiding unnecessary downtime and ensuring that equipment is serviced precisely when required. Predictive maintenance allows for more efficient resource allocation, reducing disruptions to production cycles and operational costs.
Case Study : General Electric | Industry: Energy
· Challenge: GE’s power generation turbines required regular maintenance, but the traditional schedule was based on time intervals, which frequently resulted in unnecessary maintenance or unplanned breakdowns.
· Solution: GE created an AI-powered system that analyzes sensor data from turbines to predict the best time for maintenance.
· Benefits:
o Increased turbine uptime by 10%
o Reduced maintenance costs by 20%
o Improved reliability and efficiency of power generation
Notification for Maintenance Needs
AI-powered systems continuously track equipment performance and health indicators. When anomalies or signs of potential problems are detected, these systems issue alerts or notifications for necessary maintenance interventions. This proactive notification system enables prompt action, preventing equipment failures and ensuring operational continuity.
Case Study: United Parcel Service (UPS) | Industry: Logistics
· Challenge: UPS’s delivery vehicles were prone to breakdowns, disrupting delivery schedules and costing the company money.
· Solution: UPS implemented an AI-powered system that analyzed vehicle data in real time to detect potential issues and alert mechanics to the need for preventive maintenance.
· Benefits:
o Reduced breakdowns by 20%
o Improved on-time delivery rates
o Saved millions of dollars in maintenance costs
Detection of Probable Faults
AI’s ability to sift through massive amounts of data allows for the detection of subtle patterns that may indicate impending faults. Machine learning algorithms can learn from historical data to identify early signs of equipment degradation or malfunction, allowing for proactive measures to address issues before they worsen.
Case Study : Siemens | Industry: Manufacturing
· Challenge: Siemens’ manufacturing plants were prone to equipment failures, resulting in production delays and quality issues.
· Solution: Siemens implemented an AI-powered system that monitored sensors in equipment to detect early signs of wear and tear and predict potential failure.
· Benefits:
o Reduced equipment downtime by 15%
o Improved product quality
o Reduced production costs
Scheduled Notifications
AI systems can generate automated notifications or work orders for maintenance tasks based on predefined parameters or detected anomalies. These notifications ensure that maintenance activities are scheduled and carried out at the optimal times, minimizing disruptions to ongoing operations.
Case Study : John Deere | Industry: Agriculture
· Challenge: Farmers frequently struggled to determine when to service their John Deere tractors, which could result in costly breakdowns.
· Solution: John Deere created an AI-powered system that analyzes tractor data and alerts farmers to the need for maintenance based on actual usage.
· Benefits:
o Reduced tractor downtime by 10%
o Saved farmers money on maintenance costs
o Improved productivity and yield
Production of Machine Health Reports
AI systems generate comprehensive reports on machine health, providing detailed information about equipment performance, historical trends, and potential risks. These reports facilitate decision-making by providing actionable information for improving maintenance strategies, allocating resources effectively, and planning for equipment upgrades or replacements.
Case Study : Amazon | Industry: Retail
· Challenge: Amazon’s data centers were critical to its operations, but monitoring and maintaining the equipment’s health proved difficult.
· Solution: Amazon created an AI-powered system that collected and analyzed data from data center equipment in real time, generating health reports and predicting potential failures.
· Benefits:
o Reduced data center downtime by 25%
o Improved data center efficiency
o Saved millions of dollars in operational costs
The incorporation of AI into predictive management not only improves maintenance operations, but also shifts businesses from reactive to proactive maintenance models. Companies can gain a competitive advantage by leveraging AI to improve operational efficiency, reduce costs, and ensure critical equipment continues to function properly.
As AI technology advances, businesses across industries should leverage its capabilities to improve predictive management strategies. Adopting AI-driven predictive analytics fosters a culture of foresight, allowing businesses to stay ahead of maintenance needs, reduce downtime, and ultimately drive long-term growth and success.
1 thought on “AI In Business & Predictive Management”